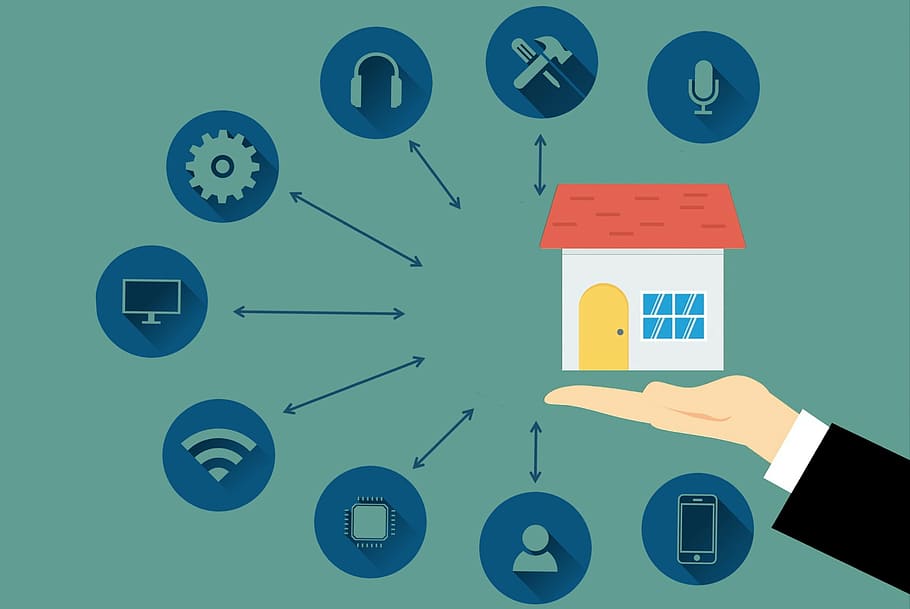
Real Estate Data Mining
What is Data Mining for Real Estate?
Companies large and small are now more interested in consumer data. Where people go, what they buy and the level of satisfaction are all important metrics in the 21st century. In the housing industry, a range of data is available to investors if they know where to find it. Asking the question what is data mining for real estate is a good way to learn this secret.
Definition of Data Mining
In the computer world, programmers and annalists earn big salaries for creating ways to track consumer activities. Companies that have a source of useable data can usually outperform competition. Companies like Google, eBay, Facebook and Twitter all participate in some version of data mining in the global market.
Real Estate Data Mining for Investors
Agents, brokers and financial institutions all take an interest in consumer behavior. Employment history, martial status, values of properties and recent homes sales all help create usable data for real estate companies. Investors can now use this data in order to find ways to buy houses in markets that are performing at high percentages.
Types of Mined Data Available:
1. Bank-Owned Real Estate (REO)
2. Wholesale Sellers
3. Short Sale Listings
4. New Builders
5. Real Estate Auctions
6. Updated Foreclosure Listings
7. FSBO Lists (For Sale by Owner)
8. Divorce Finalizations
9. Bankruptcy Filings
10. Probate Homes for Sale
How Data Mining Helps Uncover Deals
One key component that all successful real estate entrepreneurs have available is accurate information. He or she who has good data will almost always come out on top. Because deals come and go on a daily basis, being the first to capitalize on a market deal can benefit an investor.
Someone who wants to review current home prices in a given area can use the tools provided by, or While these are usually retail price homes, it can be helpful to a person who plans to invest in a specific neighborhood to understand the economic conditions.
Savvy investors must use more than one resource to find homes for sale in any market. While a smaller portion of people plan to flip homes for a profit, other people buy and hold real estate in hopes of earning a much larger profit in the future. Having access to mined data and knowing how to put the data to use is a big plus for any real estate investing professional.
Renovated Houses for Sale in Florida
Investors who do not have the time or resources to commit to putting data mining to use in the housing industry can purchase managed investment homes from JWB in Florida. Annual ROI as high as 15 percent is now part of investor packages showcased in the turnkey guide offered as a free download on this website.
I am a co-founder at JWB Real Estate Capital, and I love to talk about investing in rental properties! You’ll often find me here contributing to our blog and in our Facebook group connecting with the community & sharing insights.
Mapping of Real Estate Prices Using Data Mining Techniques
View PDFUnder a Creative Commons licenseopen accessAbstractThe paper describes an innovative software that is used for real estate evaluation and mapping and analyzing of real estate advertisements published on the internet in the Czech Republic. The software systematically collects, analyzes and assesses data about the changes in the real estate market. For each half year, the software assembles over 650, 000 price quotations concerning sale or rental of apartments, houses, business properties and building lots. All real estate advertisements are continuously stored in a software database and are thoroughly analyzed for their have been numerous articles concerning real estate market analysis in both mass media and scholarly publications. Unfortunately, not all presented information is objective and unbiased. Many cases by “independent specialists” have stated information with no verified research. We have witnessed manipulation of information by lobbies (such as banks offering mortgage agents, real estate companies and agents, building companies, developers, majority owners, etc. ). The author of this paper offers objective and unbiased evaluation of price development in real estate market. The author brings forward information based on extensive research and large amounts of statistical data which has been collected continuously from year 2007 until ywordsdata miningevaluation of propertiesreal estate marketsoftwarestatistical pyright © 2015 The Authors. Published by Elsevier Ltd.
Data Mining Techniques: Types of Data, Methods, Applications – upGrad
Home > Data Science > Data Mining Techniques: Types of Data, Methods, Applications
Businesses these days are collecting data at a very striking rate. The sources of this enormous data stream are varied. It could come from credit card transactions, publicly available customer data, data from banks and financial institutions, as well as the data that users have to provide just to use and download an application on their laptops, mobile phones, tablets, and desktops.
It is not easy to store such massive amounts of data. So, many relational database servers are being continuously built for this purpose. Online transactional protocol or OLTP systems are also being developed to store all that into different database servers. OLTP systems play a vital role in helping businesses function smoothly.
It is these systems that are responsible for storing data that comes out of the smallest of transactions into the database. So, data related to sale, purchase, human capital management, and other transactions are stored in database servers by OLTP systems.
Now, top executives need access to facts based on data to base their decisions on. This is where online analytical processing or OLAP systems enter the picture. Data warehouses and other OLAP systems are built more and more because of this very need of or top executives. We don’t only need data but also the analytics associated with it to make better and more profitable decisions. OLTP and OLAP systems work in tandem.
OLTP systems store all massive amounts of data that we generate on a daily basis. This data is then sent to OLAP systems for building data-based analytics. If you don’t already know, then let us tell you that data plays a very important role in the growth of a company. It can help in making knowledge-backed decisions that can take a company to the next level of growth. Data examination should never happen superficially.
It doesn’t serve the purpose. We need to analyze data to enrich ourselves with the knowledge that will help us in making the right calls for the success of our business. All the data that we have been flooded with these days isn’t of any use if we aren’t learning anything from it. Data available to us is so huge that it is humanly impossible for us to process it and make sense of it. Data mining or knowledge discovery is what we need to solve this problem. Learn about other applications of data mining in real world.
What is Data Mining? Data Mining ProcessTypes of data that can be mined1. Data stored in the database2. Data warehouse3. Transactional data4. Other types of dataData Mining Techniques1. Association2. Clustering3. Classification4. Prediction5. Sequential patternsData Mining Applications1. Healthcare2. Education3. Market basket analysis4. Customer relationship management (CRM)5. Manufacturing engineering6. Finance and banking7. Fraud detection8. Monitoring Patterns9. ClassificationBased on the type of data sources, minedBased on the data framework involvedBased on data mining functionalitiesBased on user interaction in data mining10. Association11. Anomaly Detection12. Clustering13. Regression14. Prediction15. Sequential Patterns16. Decision Trees17. Visualization18. Neural Networks19. Data WarehousingData Mining ToolsConclusion
What is Data Mining?
Data mining is the process that helps in extracting information from a given data set to identify trends, patterns, and useful data. The objective of using data mining is to make data-supported decisions from enormous data sets.
Data mining works in conjunction with predictive analysis, a branch of statistical science that uses complex algorithms designed to work with a special group of problems. The predictive analysis first identifies patterns in huge amounts of data, which data mining generalizes for predictions and forecasts. Data mining serves a unique purpose, which is to recognize patterns in datasets for a set of problems that belong to a specific domain.
It does this by using a sophisticated algorithm to train a model for a specific problem. When you know the domain of the problem you are dealing with, you can even use machine learning to model a system that is capable of identifying patterns in a data set. When you put machine learning to work, you will be automating the problem-solving system as a whole, and you wouldn’t need to come up with special programming to solve every problem that you come across.
We can also define data mining as a technique of investigation patterns of data that belong to particular perspectives. This helps us in categorizing that data into useful information. This useful information is then accumulated and assembled to either be stored in database servers, like data warehouses, or used in data mining algorithms and analysis to help in decision making. Moreover, it can be used for revenue generation and cost-cutting amongst other purposes.
Data mining is the process of searching large sets of data to look out for patterns and trends that can’t be found using simple analysis techniques. It makes use of complex mathematical algorithms to study data and then evaluate the possibility of events happening in the future based on the findings. It is also referred to as knowledge discovery of data or KDD.
Data mining is used by businesses to draw out specific information from large volumes of data to find solutions to their business problems. It has the capability of transforming raw data into information that can help businesses grow by taking better decisions. Data mining has several types, including pictorial data mining, text mining, social media mining, web mining, and audio and video mining amongst others.
Read: Data Mining vs Machine Learning
Data Mining Process
Before the actual data mining could occur, there are several processes involved in data mining implementation. Here’s how:
Step 1: Business Research – Before you begin, you need to have a complete understanding of your enterprise’s objectives, available resources, and current scenarios in alignment with its requirements. This would help create a detailed data mining plan that effectively reaches organizations’ goals.
Step 2: Data Quality Checks – As the data gets collected from various sources, it needs to be checked and matched to ensure no bottlenecks in the data integration process. The quality assurance helps spot any underlying anomalies in the data, such as missing data interpolation, keeping the data in top-shape before it undergoes mining.
Step 3: Data Cleaning – It is believed that 90% of the time gets taken in the selecting, cleaning, formatting, and anonymizing data before mining.
Step 4: Data Transformation – Comprising five sub-stages, here, the processes involved make data ready into final data sets. It involves:
Data Smoothing: Here, noise is removed from the data.
Data Summary: The aggregation of data sets is applied in this process.
Data Generalization: Here, the data gets generalized by replacing any low-level data with higher-level conceptualizations.
Data Normalization: Here, data is defined in set ranges.
Data Attribute Construction: The data sets are required to be in the set of attributes before data mining.
Step 5: Data Modelling: For better identification of data patterns, several mathematical models are implemented in the dataset, based on several conditions. Learn data science to understand and utilize the power of data mining.
Types of data that can be mined
1. Data stored in the database
A database is also called a database management system or DBMS. Every DBMS stores data that are related to each other in a way or the other. It also has a set of software programs that are used to manage data and provide easy access to it. These software programs serve a lot of purposes, including defining structure for database, making sure that the stored information remains secured and consistent, and managing different types of data access, such as shared, distributed, and concurrent.
A relational database has tables that have different names, attributes, and can store rows or records of large data sets. Every record stored in a table has a unique key. Entity-relationship model is created to provide a representation of a relational database that features entities and the relationships that exist between them.
2. Data warehouse
A data warehouse is a single data storage location that collects data from multiple sources and then stores it in the form of a unified plan. When data is stored in a data warehouse, it undergoes cleaning, integration, loading, and refreshing. Data stored in a data warehouse is organized in several parts. If you want information on data that was stored 6 or 12 months back, you will get it in the form of a summary.
3. Transactional data
Transactional database stores record that are captured as transactions. These transactions include flight booking, customer purchase, click on a website, and others. Every transaction record has a unique ID. It also lists all those items that made it a transaction.
4. Other types of data
We have a lot of other types of data as well that are known for their structure, semantic meanings, and versatility. They are used in a lot of applications. Here are a few of those data types: data streams, engineering design data, sequence data, graph data, spatial data, multimedia data, and more.
Data Mining Techniques
1. Association
It is one of the most used data mining techniques out of all the others. In this technique, a transaction and the relationship between its items are used to identify a pattern. This is the reason this technique is also referred to as a relation technique. It is used to conduct market basket analysis, which is done to find out all those products that customers buy together on a regular basis.
This technique is very helpful for retailers who can use it to study the buying habits of different customers. Retailers can study sales data of the past and then lookout for products that customers buy together. Then they can put those products in close proximity of each other in their retail stores to help customers save their time and to increase their sales.
2. Clustering
This technique creates meaningful object clusters that share the same characteristics. People often confuse it with classification, but if they properly understand how both these techniques work, they won’t have any issue. Unlike classification that puts objects into predefined classes, clustering puts objects in classes that are defined by it.
Let us take an example. A library is full of books on different topics. Now the challenge is to organize those books in a way that readers don’t have any problem in finding out books on a particular topic. We can use clustering to keep books with similarities in one shelf and then give those shelves a meaningful name. Readers looking for books on a particular topic can go straight to that shelf. They won’t be required to roam the entire library to find their book.
3. Classification
This technique finds its origins in machine learning. It classifies items or variables in a data set into predefined groups or classes. It uses linear programming, statistics, decision trees, and artificial neural network in data mining, amongst other techniques. Classification is used to develop software that can be modelled in a way that it becomes capable of classifying items in a data set into different classes.
For instance, we can use it to classify all the candidates who attended an interview into two groups – the first group is the list of those candidates who were selected and the second is the list that features candidates that were rejected. Data mining software can be used to perform this classification job.
4. Prediction
This technique predicts the relationship that exists between independent and dependent variables as well as independent variables alone. It can be used to predict future profit depending on the sale. Let us assume that profit and sale are dependent and independent variables, respectively. Now, based on what the past sales data says, we can make a profit prediction of the future using a regression curve.
5. Sequential patterns
This technique aims to use transaction data, and then identify similar trends, patterns, and events in it over a period of time. The historical sales data can be used to discover items that buyers bought together at different times of the year. Business can make sense of this information by recommending customers to buy those products at times when the historical data doesn’t suggest they would. Businesses can use lucrative deals and discounts to push through this recommendation.
Data Mining Applications
Below are some most useful data mining applications lets know more about them.
1. Healthcare
Data mining has the potential to transform the healthcare system completely. It can be used to identify best practices based on data and analytics, which can help healthcare facilities to reduce costs and improve patient outcomes. Data mining, along with machine learning, statistics, data visualization, and other techniques can be used to make a difference. It can come in handy when forecasting patients of different categories. This will help patients to receive intensive care when and where they want it. Data mining can also help healthcare insurers to identify fraudulent activities.
2. Education
Use of data mining in education is still in its nascent phase. It aims to develop techniques that can use data coming out of education environments for knowledge exploration. The purposes that these techniques are expected to serve include studying how educational support impacts students, supporting the future-leaning needs of students, and promoting the science of learning amongst others. Educational institutions can use these techniques to not only predict how students are going to do in examinations but also make accurate decisions. With this knowledge, these institutions can focus more on their teaching pedagogy.
3. Market basket analysis
This is a modelling technique that uses hypothesis as a basis. The hypothesis says that if you purchase certain products, then it is highly likely that you will also purchase products that don’t belong to that group that you usually purchase from. Retailers can use this technique to understand the buying habits of their customers. Retailers can use this information to make changes in the layout of their store and to make shopping a lot easier and less time consuming for customers.
4. Customer relationship management (CRM)
CRM involves acquiring and keeping customers, improving loyalty, and employing customer-centric strategies. Every business needs customer data to analyze it and use the findings in a way that they can build a long-lasting relationship with their customers. Data mining can help them do that.
5. Manufacturing engineering
A manufacturing company relies a lot on the data or information available to it. Data mining can help these companies in identifying patterns in processes that are too complex for a human mind to understand. They can identify the relationships that exist between different system-level designing elements, including customer data needs, architecture, and portfolio of products.
Data mining can also prove useful in forecasting the overall time required for product development, the cost involved in the process, and the expectations companies can have from the final product.
6. Finance and banking
The banking system has been witnessing the generation of massive amounts of data from the time it underwent digitalization. Bankers can use data mining techniques to solve the baking and financial problems that businesses face by finding out correlations and trends in market costs and business information. This job is too difficult without data mining as the volume of data that they are dealing with is too large. Managers in the banking and financial sectors can use this information to acquire, retain, and maintain a customer.
Learn more: Association Rule Mining
7. Fraud detection
Fraudulent activities cost businesses billions of dollars every year. Methods that are usually used for detecting frauds are too complex and time-consuming. Data mining provides a simple alternative. Every ideal fraud detection system needs to protect user data in all circumstances. A method is supervised to collect data, and then this data is categorized into fraudulent or non-fraudulent data. This data is used in training a model that identifies every document as fraudulent or non-fraudulent.
8. Monitoring Patterns
Known as one of the fundamental data mining techniques, it generally comprises tracking data patterns to derive business conclusions. For an organization, it could mean anything from identifying sales upsurge or tapping newer demographics.
9. Classification
To derive relevant metadata, the classification technique in data mining helps in differentiating data into separate classes:
Based on the type of data sources, mined
Depending on the type of data handled like text-based data, multimedia data, spatial data, time-series data, etc.
Based on the data framework involved
Any data set that is based on the object-oriented database, relational database, etc.
Based on data mining functionalities
Here the data sets are differentiated based on the approach taken like Machine Learning, Algorithms, Statistics, Database or data warehouse, etc.
Based on user interaction in data mining
The datasets are used to differentiate based on query-driven systems, autonomous systems.
10. Association
Otherwise known as relation technique, the data is identified based on the relationship between the values in the same transaction. It is especially handy for organizations trying to spot trends into purchases or product preferences. Since it is related to customers’ shopping behavior, an organization can break down data patterns based on the buyers’ purchase histories.
11. Anomaly Detection
If a data item is identified that does not match up to a precedent behavior, it is an outlier or an exception. This method digs deep into the process of the creation of such exceptions and backs it with critical information.
Generally, anomalies can be aloof in its origin, but it also comes with the possibility of finding out a focus area. Therefore, businesses often use this method to trace system intrusion, error detection, and keeping a check on the system’s overall health. Experts prefer the emission of anomalies from the data sets to increase the chances of correctness.
12. Clustering
Just as it sounds, this technique involves collating identical data objects into the same clusters. Based on the dissimilarities, the groups often consist of using metrics to facilitate maximum data association. Such processes can be helpful to profile customers based on their income, shopping frequency, etc.
Check out: Difference between Data Science and Data Mining
13. Regression
A data mining process that helps in predicting customer behavior and yield, it is used by enterprises to understand the correlation and independence of variables in an environment. For product development, such analysis can help understand the influence of factors like market demands, competition, etc.
14. Prediction
As implied in its name, this compelling data mining technique helps enterprises to match patterns based on current and historical data records for predictive analysis of the future. While some of the approaches involve Artificial Intelligence and Machine Learning aspects, some can be conducted via simple algorithms.
Organizations can often predict profits, derive regression values, and more with such data mining techniques.
15. Sequential Patterns
It is used to identify striking patterns, trends in the transaction data available in the given time. For discovering items that customers prefer to buy at different times of the year, businesses offer deals on such products.
Read: Data Mining Project Ideas
16. Decision Trees
One of the most commonly used data mining techniques; here, a simple condition is the crux of the method. Since such terms have multiple answers, each of the solutions further branches out into more states until the conclusion is reached. Learn more about decision trees.
17. Visualization
No data is useful without visualizing the right way since it’s always changing. The different colors and objects can reveal valuable trends, patterns, and insights into the vast datasets. Therefore, businesses often turn to data visualization dashboards that automate the process of generating numerical models.
18. Neural Networks
It represents the connection of a particular machine learning model to an AI-based learning technique. Since it is inspired by the neural multi-layer system found in human anatomy, it represents the working of machine learning models in precision. It can be increasingly complex and therefore needs to be dealt with extreme care.
19. Data Warehousing
While it means data storage, it symbolizes the storing of data in the form of cloud warehouses. Companies often use such a precise data mining method to have more in-depth real-time data analysis. Read more about data warehousing.
Data Mining Tools
All that AI and Machine learning inference must have got you into wondering that for data mining implementation, you’d require nothing less. That might not entirely be true, as, with the help of most straightforward databases, you can get the job done with equal accuracy.
Also, read about the most useful data mining applications.
Conclusion
Data mining brings together different methods from a variety of disciplines, including data visualization, machine learning, database management, statistics, and others. These techniques can be made to work together to tackle complex problems. Generally, data mining software or systems make use of one or more of these methods to deal with different data requirements, types of data, application areas, and mining tasks.
If you are curious to learn about data science, check out IIIT-B & upGrad’s Executive PG Programme in Data Science which is created for working professionals and offers 10+ case studies & projects, practical hands-on workshops, mentorship with industry experts, 1-on-1 with industry mentors, 400+ hours of learning and job assistance with top firms.
Prepare for a Career of the Future
LEARN FROM INDUSTRY-RELEVANT CURRICULUM, TOP EXPERTS & FACULTY.
Apply Now
Frequently Asked Questions about real estate data mining
What are the 3 types of data mining?
Data mining has several types, including pictorial data mining, text mining, social media mining, web mining, and audio and video mining amongst others.Read: Data Mining vs Machine Learning.Learn more: Association Rule Mining.Check out: Difference between Data Science and Data Mining.Read: Data Mining Project Ideas.Apr 30, 2020
What is scraping in real estate?
Scraping real estate listings would mean setting up web crawlers to scrape the desired data points held in real estate websites and other sources like digital classifieds. The bots would fetch this data and the information will be transformed into a structured format that enables analytics.
Where does real estate data come from?
Real estate data is collected from both public and commercial records that can be found via municipal, realtor, and broker websites or archives.