…
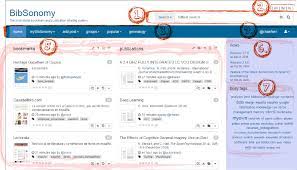
Python 3 Web Crawler
Crawling and Scraping Web Pages with Scrapy and Python 3
Introduction
Web scraping, often called web crawling or web spidering, or “programmatically going over a collection of web pages and extracting data, ” is a powerful tool for working with data on the web.
With a web scraper, you can mine data about a set of products, get a large corpus of text or quantitative data to play around with, get data from a site without an official API, or just satisfy your own personal curiosity.
In this tutorial, you’ll learn about the fundamentals of the scraping and spidering process as you explore a playful data set. We’ll use BrickSet, a community-run site that contains information about LEGO sets. By the end of this tutorial, you’ll have a fully functional Python web scraper that walks through a series of pages on Brickset and extracts data about LEGO sets from each page, displaying the data to your screen.
The scraper will be easily expandable so you can tinker around with it and use it as a foundation for your own projects scraping data from the web.
Prerequisites
To complete this tutorial, you’ll need a local development environment for Python 3. You can follow How To Install and Set Up a Local Programming Environment for Python 3 to configure everything you need.
Step 1 — Creating a Basic Scraper
Scraping is a two step process:
You systematically find and download web pages.
You take those web pages and extract information from them.
Both of those steps can be implemented in a number of ways in many languages.
You can build a scraper from scratch using modules or libraries provided by your programming language, but then you have to deal with some potential headaches as your scraper grows more complex. For example, you’ll need to handle concurrency so you can crawl more than one page at a time. You’ll probably want to figure out how to transform your scraped data into different formats like CSV, XML, or JSON. And you’ll sometimes have to deal with sites that require specific settings and access patterns.
You’ll have better luck if you build your scraper on top of an existing library that handles those issues for you. For this tutorial, we’re going to use Python and Scrapy to build our scraper.
Scrapy is one of the most popular and powerful Python scraping libraries; it takes a “batteries included” approach to scraping, meaning that it handles a lot of the common functionality that all scrapers need so developers don’t have to reinvent the wheel each time. It makes scraping a quick and fun process!
Scrapy, like most Python packages, is on PyPI (also known as pip). PyPI, the Python Package Index, is a community-owned repository of all published Python software.
If you have a Python installation like the one outlined in the prerequisite for this tutorial, you already have pip installed on your machine, so you can install Scrapy with the following command:
pip install scrapy
If you run into any issues with the installation, or you want to install Scrapy without using pip, check out the official installation docs.
With Scrapy installed, let’s create a new folder for our project. You can do this in the terminal by running:
mkdir brickset-scraper
Now, navigate into the new directory you just created:
cd brickset-scraper
Then create a new Python file for our scraper called We’ll place all of our code in this file for this tutorial. You can create this file in the terminal with the touch command, like this:
touch
Or you can create the file using your text editor or graphical file manager.
We’ll start by making a very basic scraper that uses Scrapy as its foundation. To do that, we’ll create a Python class that subclasses, a basic spider class provided by Scrapy. This class will have two required attributes:
name — just a name for the spider.
start_urls — a list of URLs that you start to crawl from. We’ll start with one URL.
Open the file in your text editor and add this code to create the basic spider:
import scrapy
class BrickSetSpider():
name = “brickset_spider”
start_urls = [”]
Let’s break this down line by line:
First, we import scrapy so that we can use the classes that the package provides.
Next, we take the Spider class provided by Scrapy and make a subclass out of it called BrickSetSpider. Think of a subclass as a more specialized form of its parent class. The Spider subclass has methods and behaviors that define how to follow URLs and extract data from the pages it finds, but it doesn’t know where to look or what data to look for. By subclassing it, we can give it that information.
Then we give the spider the name brickset_spider.
Finally, we give our scraper a single URL to start from:. If you open that URL in your browser, it will take you to a search results page, showing the first of many pages containing LEGO sets.
Now let’s test out the scraper. You typically run Python files by running a command like python path/to/ However, Scrapy comes with its own command line interface to streamline the process of starting a scraper. Start your scraper with the following command:
scrapy runspider
You’ll see something like this:
Output2016-09-22 23:37:45 [scrapy] INFO: Scrapy 1. 1. 2 started (bot: scrapybot)
2016-09-22 23:37:45 [scrapy] INFO: Overridden settings: {}
2016-09-22 23:37:45 [scrapy] INFO: Enabled extensions:
[‘scrapy. extensions. logstats. LogStats’,
”,
‘reStats’]
2016-09-22 23:37:45 [scrapy] INFO: Enabled downloader middlewares:
[‘tpAuthMiddleware’,…
”]
2016-09-22 23:37:45 [scrapy] INFO: Enabled spider middlewares:
[‘tpErrorMiddleware’,…
2016-09-22 23:37:45 [scrapy] INFO: Enabled item pipelines:
[]
2016-09-22 23:37:45 [scrapy] INFO: Spider opened
2016-09-22 23:37:45 [scrapy] INFO: Crawled 0 pages (at 0 pages/min), scraped 0 items (at 0 items/min)
2016-09-22 23:37:45 [scrapy] DEBUG: Telnet console listening on 127. 0. 1:6023
2016-09-22 23:37:47 [scrapy] DEBUG: Crawled (200)
2016-09-22 23:37:47 [scrapy] INFO: Closing spider (finished)
2016-09-22 23:37:47 [scrapy] INFO: Dumping Scrapy stats:
{‘downloader/request_bytes’: 224,
‘downloader/request_count’: 1,…
‘scheduler/enqueued/memory’: 1,
‘start_time’: time(2016, 9, 23, 6, 37, 45, 995167)}
2016-09-22 23:37:47 [scrapy] INFO: Spider closed (finished)
That’s a lot of output, so let’s break it down.
The scraper initialized and loaded additional components and extensions it needed to handle reading data from URLs.
It used the URL we provided in the start_urls list and grabbed the HTML, just like your web browser would do.
It passed that HTML to the parse method, which doesn’t do anything by default. Since we never wrote our own parse method, the spider just finishes without doing any work.
Now let’s pull some data from the page.
We’ve created a very basic program that pulls down a page, but it doesn’t do any scraping or spidering yet. Let’s give it some data to extract.
If you look at the page we want to scrape, you’ll see it has the following structure:
There’s a header that’s present on every page.
There’s some top-level search data, including the number of matches, what we’re searching for, and the breadcrumbs for the site.
Then there are the sets themselves, displayed in what looks like a table or ordered list. Each set has a similar format.
When writing a scraper, it’s a good idea to look at the source of the HTML file and familiarize yourself with the structure. So here it is, with some things removed for readability:
…
Scraping this page is a two step process:
First, grab each LEGO set by looking for the parts of the page that have the data we want.
Then, for each set, grab the data we want from it by pulling the data out of the HTML tags.
scrapy grabs data based on selectors that you provide. Selectors are patterns we can use to find one or more elements on a page so we can then work with the data within the element. scrapy supports either CSS selectors or XPath selectors.
We’ll use CSS selectors for now since CSS is the easier option and a perfect fit for finding all the sets on the page. If you look at the HTML for the page, you’ll see that each set is specified with the class set. Since we’re looking for a class, we’d use for our CSS selector. All we have to do is pass that selector into the response object, like this:
def parse(self, response):
SET_SELECTOR = ”
for brickset in (SET_SELECTOR):
pass
This code grabs all the sets on the page and loops over them to extract the data. Now let’s extract the data from those sets so we can display it.
Another look at the source of the page we’re parsing tells us that the name of each set is stored within an h1 tag for each set:
Brick Bank
10251-1
The brickset object we’re looping over has its own css method, so we can pass in a selector to locate child elements. Modify your code as follows to locate the name of the set and display it:
NAME_SELECTOR = ‘h1::text’
yield {
‘name’: (NAME_SELECTOR). extract_first(), }
Note: The trailing comma after extract_first() isn’t a typo. We’re going to add more to this section soon, so we’ve left the comma there to make adding to this section easier later.
You’ll notice two things going on in this code:
We append::text to our selector for the name. That’s a CSS pseudo-selector that fetches the text inside of the a tag rather than the tag itself.
We call extract_first() on the object returned by (NAME_SELECTOR) because we just want the first element that matches the selector. This gives us a string, rather than a list of elements.
Save the file and run the scraper again:
This time you’ll see the names of the sets appear in the output:
Output…
[scrapy] DEBUG: Scraped from <200 >
{‘name’: ‘Brick Bank’}
{‘name’: ‘Volkswagen Beetle’}
{‘name’: ‘Big Ben’}
{‘name’: ‘Winter Holiday Train’}…
Let’s keep expanding on this by adding new selectors for images, pieces, and miniature figures, or minifigs that come with a set.
Take another look at the HTML for a specific set:
…
The brickset object we’re looping over has its own css method, so we can pass in a selector to locate child elements. Modify your code as follows to locate the name of the set and display it:
NAME_SELECTOR = ‘h1::text’
yield {
‘name’: (NAME_SELECTOR). extract_first(), }
Note: The trailing comma after extract_first() isn’t a typo. We’re going to add more to this section soon, so we’ve left the comma there to make adding to this section easier later.
You’ll notice two things going on in this code:
We append::text to our selector for the name. That’s a CSS pseudo-selector that fetches the text inside of the a tag rather than the tag itself.
We call extract_first() on the object returned by (NAME_SELECTOR) because we just want the first element that matches the selector. This gives us a string, rather than a list of elements.
Save the file and run the scraper again:
This time you’ll see the names of the sets appear in the output:
Output…
[scrapy] DEBUG: Scraped from <200 >
{‘name’: ‘Brick Bank’}
{‘name’: ‘Volkswagen Beetle’}
{‘name’: ‘Big Ben’}
{‘name’: ‘Winter Holiday Train’}…
Let’s keep expanding on this by adding new selectors for images, pieces, and miniature figures, or minifigs that come with a set.
Take another look at the HTML for a specific set:
10251: Brick Bank
…
We can see a few things by examining this code:
The image for the set is stored in the src attribute of an img tag inside an a tag at the start of the set. We can use another CSS selector to fetch this value just like we did when we grabbed the name of each set.
Getting the number of pieces is a little trickier. There’s a dt tag that contains the text Pieces, and then a dd tag that follows it which contains the actual number of pieces. We’ll use XPath, a query language for traversing XML, to grab this, because it’s too complex to be represented using CSS selectors.
Getting the number of minifigs in a set is similar to getting the number of pieces. There’s a dt tag that contains the text Minifigs, followed by a dd tag right after that with the number.
So, let’s modify the scraper to get this new information:
name = ‘brick_spider’
PIECES_SELECTOR = ‘. //dl[dt/text() = “Pieces”]/dd/a/text()’
MINIFIGS_SELECTOR = ‘. //dl[dt/text() = “Minifigs”]/dd[2]/a/text()’
IMAGE_SELECTOR = ‘img::attr(src)’
‘name’: (NAME_SELECTOR). extract_first(),
‘pieces’: (PIECES_SELECTOR). extract_first(),
‘minifigs’: (MINIFIGS_SELECTOR). extract_first(),
‘image’: (IMAGE_SELECTOR). extract_first(), }
Save your changes and run the scraper again:
Now you’ll see that new data in the program’s output:
Output2016-09-22 23:52:37 [scrapy] DEBUG: Scraped from <200 >
{‘minifigs’: ‘5’, ‘pieces’: ‘2380’, ‘name’: ‘Brick Bank’, ‘image’: ”}
2016-09-22 23:52:37 [scrapy] DEBUG: Scraped from <200 >
{‘minifigs’: None, ‘pieces’: ‘1167’, ‘name’: ‘Volkswagen Beetle’, ‘image’: ”}
{‘minifigs’: None, ‘pieces’: ‘4163’, ‘name’: ‘Big Ben’, ‘image’: ”}
{‘minifigs’: None, ‘pieces’: None, ‘name’: ‘Winter Holiday Train’, ‘image’: ”}
{‘minifigs’: None, ‘pieces’: None, ‘name’: ‘XL Creative Brick Box’, ‘image’: ‘/assets/images/misc/’}
{‘minifigs’: None, ‘pieces’: ‘583’, ‘name’: ‘Creative Building Set’, ‘image’: ”}
Now let’s turn this scraper into a spider that follows links.
Step 3 — Crawling Multiple Pages
We’ve successfully extracted data from that initial page, but we’re not progressing past it to see the rest of the results. The whole point of a spider is to detect and traverse links to other pages and grab data from those pages too.
You’ll notice that the top and bottom of each page has a little right carat (>) that links to the next page of results. Here’s the HTML for that:
- …
-
Scrapy | A Fast and Powerful Scraping and Web Crawling …
An open source and collaborative framework for extracting the data you need from websites.
In a fast, simple, yet extensible way.
Maintained by
Zyte
(formerly Scrapinghub)
and
many other contributors
Install the latest version of Scrapy
Scrapy 2. 5. 0
pip install scrapy
Terminal•
cat > <
# Deploy the spider to Zyte Scrapy Cloud
shub deploy
# Schedule the spider for execution
shub schedule blogspider
Spider blogspider scheduled, watch it running here:
# Retrieve the scraped data
shub items 26731/1/8
{“title”: “Improved Frontera: Web Crawling at Scale with Python 3 Support”}
{“title”: “How to Crawl the Web Politely with Scrapy”}…
Fast and powerful
write the rules to extract the data and let Scrapy do the rest
Easily extensible
extensible by design, plug new functionality easily without having to touch the core
Portable, Python
written in Python and runs on Linux, Windows, Mac and BSD
Healthy community
– 36. 3k stars, 8. 4k forks and 1. 8k watchers on GitHub
– 5. 1k followers on Twitter
– 14. 7k questions on StackOverflow
Web crawling with Python – ScrapingBee
●
11 December, 2020
13 min read
Ari is an expert Data Engineer and a talented technical writer. He wrote the entire Scrapy integration for ScrapingBee and this awesome article.
Web crawling is a powerful technique to collect data from the web by finding all the URLs for one or multiple domains. Python has several popular web crawling libraries and frameworks.
In this article, we will first introduce different crawling strategies and use cases. Then we will build a simple web crawler from scratch in Python using two libraries: requests and Beautiful Soup. Next, we will see why it’s better to use a web crawling framework like Scrapy. Finally, we will build an example crawler with Scrapy to collect film metadata from IMDb and see how Scrapy scales to websites with several million pages.
What is a web crawler?
Web crawling and web scraping are two different but related concepts. Web crawling is a component of web scraping, the crawler logic finds URLs to be processed by the scraper code.
A web crawler starts with a list of URLs to visit, called the seed. For each URL, the crawler finds links in the HTML, filters those links based on some criteria and adds the new links to a queue. All the HTML or some specific information is extracted to be processed by a different pipeline.
Web crawling strategies
In practice, web crawlers only visit a subset of pages depending on the crawler budget, which can be a maximum number of pages per domain, depth or execution time.
Most popular websites provide a file to indicate which areas of the website are disallowed to crawl by each user agent. The opposite of the robots file is the file, that lists the pages that can be crawled.
Popular web crawler use cases include:
Search engines (Googlebot, Bingbot, Yandex Bot…) collect all the HTML for a significant part of the Web. This data is indexed to make it searchable.
SEO analytics tools on top of collecting the HTML also collect metadata like the response time, response status to detect broken pages and the links between different domains to collect backlinks.
Price monitoring tools crawl e-commerce websites to find product pages and extract metadata, notably the price. Product pages are then periodically revisited.
Common Crawl maintains an open repository of web crawl data. For example, the archive from October 2020 contains 2. 71 billion web pages.
Next, we will compare three different strategies for building a web crawler in Python. First, using only standard libraries, then third party libraries for making HTTP requests and parsing HTML and finally, a web crawling framework.
Building a simple web crawler in Python from scratch
To build a simple web crawler in Python we need at least one library to download the HTML from a URL and an HTML parsing library to extract links. Python provides standard libraries urllib for making HTTP requests and for parsing HTML. An example Python crawler built only with standard libraries can be found on Github.
The standard Python libraries for requests and HTML parsing are not very developer-friendly. Other popular libraries like requests, branded as HTTP for humans, and Beautiful Soup provide a better developer experience.
If you wan to learn more, you can check this guide about the best Python HTTP client.
You can install the two libraries locally.
A basic crawler can be built following the previous architecture diagram.
import logging
from import urljoin
import requests
from bs4 import BeautifulSoup
sicConfig(
format=’%(asctime)s%(levelname)s:%(message)s’, )
class Crawler:
def __init__(self, urls=[]):
sited_urls = []
self. urls_to_visit = urls
def download_url(self, url):
return (url)
def get_linked_urls(self, url, html):
soup = BeautifulSoup(html, ”)
for link in nd_all(‘a’):
path = (‘href’)
if path and artswith(‘/’):
path = urljoin(url, path)
yield path
def add_url_to_visit(self, url):
if url not in sited_urls and url not in self. urls_to_visit:
(url)
def crawl(self, url):
html = wnload_url(url)
for url in t_linked_urls(url, html):
d_url_to_visit(url)
def run(self):
while self. urls_to_visit:
url = (0)
(f’Crawling: {url}’)
try:
except Exception:
logging. exception(f’Failed to crawl: {url}’)
finally:
if __name__ == ‘__main__’:
Crawler(urls=[”])()
The code above defines a Crawler class with helper methods to download_url using the requests library, get_linked_urls using the Beautiful Soup library and add_url_to_visit to filter URLs. The URLs to visit and the visited URLs are stored in two separate lists. You can run the crawler on your terminal.
The crawler logs one line for each visited URL.
2020-12-04 18:10:10, 737 INFO:Crawling: 2020-12-04 18:10:11, 599 INFO:Crawling: 2020-12-04 18:10:12, 868 INFO:Crawling: 2020-12-04 18:10:13, 526 INFO:Crawling: 2020-12-04 18:10:19, 174 INFO:Crawling: 2020-12-04 18:10:20, 624 INFO:Crawling: 2020-12-04 18:10:21, 556 INFO:Crawling: The code is very simple but there are many performance and usability issues to solve before successfully crawling a complete website.
The crawler is slow and supports no parallelism. As can be seen from the timestamps, it takes about one second to crawl each URL. Each time the crawler makes a request it waits for the request to be resolved and no work is done in between.
The download URL logic has no retry mechanism, the URL queue is not a real queue and not very efficient with a high number of URLs.
The link extraction logic doesn’t support standardizing URLs by removing URL query string parameters, doesn’t handle URLs starting with #, doesn’t support filtering URLs by domain or filtering out requests to static files.
The crawler doesn’t identify itself and ignores the file.
Next, we will see how Scrapy provides all these functionalities and makes it easy to extend for your custom crawls.
Web crawling with Scrapy
Scrapy is the most popular web scraping and crawling Python framework with 40k stars on Github. One of the advantages of Scrapy is that requests are scheduled and handled asynchronously. This means that Scrapy can send another request before the previous one is completed or do some other work in between. Scrapy can handle many concurrent requests but can also be configured to respect the websites with custom settings, as we’ll see later.
Scrapy has a multi-component architecture. Normally, you will implement at least two different classes: Spider and Pipeline. Web scraping can be thought of as an ETL where you extract data from the web and load it to your own storage. Spiders extract the data and pipelines load it into the storage. Transformation can happen both in spiders and pipelines, but I recommend that you set a custom Scrapy pipeline to transform each item independently of each other. This way, failing to process an item has no effect on other items.
On top of all that, you can add spider and downloader middlewares in between components as it can be seen in the diagram below.
Scrapy Architecture Overview [source]
If you have used Scrapy before, you know that a web scraper is defined as a class that inherits from the base Spider class and implements a parse method to handle each response. If you are new to Scrapy, you can read this article for easy scraping with Scrapy.
from scrapy. spiders import Spider
class ImdbSpider(Spider):
name = ‘imdb’
allowed_domains = [”]
start_urls = [”]
def parse(self, response):
pass
Scrapy also provides several generic spider classes: CrawlSpider, XMLFeedSpider, CSVFeedSpider and SitemapSpider. The CrawlSpider class inherits from the base Spider class and provides an extra rules attribute to define how to crawl a website. Each rule uses a LinkExtractor to specify which links are extracted from each page. Next, we will see how to use each one of them by building a crawler for IMDb, the Internet Movie Database.
Building an example Scrapy crawler for IMDb
Before trying to crawl IMDb, I checked IMDb file to see which URL paths are allowed. The robots file only disallows 26 paths for all user-agents. Scrapy reads the file beforehand and respects it when the ROBOTSTXT_OBEY setting is set to true. This is the case for all projects generated with the Scrapy command startproject.
scrapy startproject scrapy_crawler
This command creates a new project with the default Scrapy project folder structure.
scrapy_crawler/
├──
└── scrapy_crawler
└── spiders
Then you can create a spider in scrapy_crawler/spiders/ with a rule to extract all links.
from scrapy. spiders import CrawlSpider, Rule
from nkextractors import LinkExtractor
class ImdbCrawler(CrawlSpider):
rules = (Rule(LinkExtractor()), )
You can launch the crawler in the terminal.
scrapy crawl imdb –logfile
You will get lots of logs, including one log for each request. Exploring the logs I noticed that even if we set allowed_domains to only crawl web pages under, there were requests to external domains, such as
2020-12-06 12:25:18 [direct] DEBUG: Redirecting (302) to <GET > from <GET [()>
IMDb redirects from URLs paths under whitelist-offsite and whitelist to external domains. There is an open Scrapy Github issue that shows that external URLs don’t get filtered out when the OffsiteMiddleware is applied before the RedirectMiddleware. To fix this issue, we can configure the link extractor to deny URLs starting with two regular expressions.
rules = (
Rule(LinkExtractor(
deny=[
(”),
(”), ], )), )
Rule and LinkExtractor classes support several arguments to filter out URLs. For example, you can ignore specific URL extensions and reduce the number of duplicate URLs by sorting query strings. If you don’t find a specific argument for your use case you can pass a custom function to process_links in LinkExtractor or process_values in Rule.
For example, IMDb has two different URLs with the same content.
To limit the number of crawled URLs, we can remove all query strings from URLs with the url_query_cleaner function from the w3lib library and use it in process_links.
from import url_query_cleaner
def process_links(links):
for link in links:
= url_query_cleaner()
yield link
(”), ], ), process_links=process_links), )
Now that we have limited the number of requests to process, we can add a parse_item method to extract data from each page and pass it to a pipeline to store it. For example, we can either extract the whole to process it in a different pipeline or select the HTML metadata. To select the HTML metadata in the header tag we can code our own XPATHs but I find it better to use a library, extruct, that extracts all metadata from an HTML page. You can install it with pip install extract.
import re
import extruct
Rule(
LinkExtractor(
(”), ], ),
process_links=process_links,
callback=’parse_item’,
follow=True), )
def parse_item(self, response):
return {
‘url’:,
‘metadata’: extruct. extract(,,
syntaxes=[‘opengraph’, ‘json-ld’]), }
I set the follow attribute to True so that Scrapy still follows all links from each response even if we provided a custom parse method. I also configured extruct to extract only Open Graph metadata and JSON-LD, a popular method for encoding linked data using JSON in the Web, used by IMDb. You can run the crawler and store items in JSON lines format to a file.
scrapy crawl imdb –logfile -o -t jsonlines
The output file contains one line for each crawled item. For example, the extracted Open Graph metadata for a movie taken from the tags in the HTML looks like this.
{
“url”: “,
“metadata”: {“opengraph”: [{
“namespace”: {“og”: “},
“properties”: [
[“og:url”, “],
[“og:image”, “],
[“og:type”, “_show”],
[“og:title”, “Peaky Blinders (TV Series 2013\u2013) – IMDb”],
[“og:site_name”, “IMDb”],
[“og:description”, “Created by Steven Knight. With Cillian Murphy, Paul Anderson, Helen McCrory, Sophie Rundle. A gangster family epic set in 1900s England, centering on a gang who sew razor blades in the peaks of their caps, and their fierce boss Tommy Shelby. “]]}]}}
The JSON-LD for a single item is too long to be included in the article, here is a sample of what Scrapy extracts from the