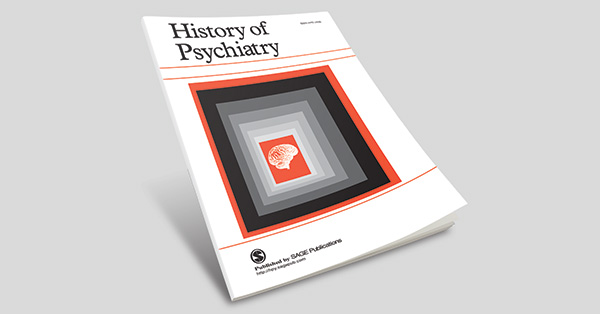
Data Extraction Tools
Top 7 Data Extraction Tools in the Market for 2021 [Hand-picked]
Home > Data Science > Top 7 Data Extraction Tools in the Market for 2021 [Hand-picked]
As the industrial world continues to bask in the glory of Data Science and Big Data, the importance of data is only strengthening and solidifying in the real world. Today, practically every major industry leverages data to gain meaningful industry insights and promote data-driven decision making for businesses. Applications of data science are increasing every day.
In such a scenario, Data Extraction becomes all the more important. The first step to leveraging data begins with data extraction from multiple and disparate sources and then comes the processing and analyzing part.
In this post, we will focus on Data Extraction and talk about some of the best Data Extraction tools available out there!
What is Data Extraction? Top Data Extraction Tools of 20211. 2. OutWit Hub3. Octoparse4. Web Scraper5. ParseHub6. Mailparser7. DocParserWrapping UpIn how many ways data can be extracted? What are the applications of OutWit Hub? Are data mining and data extraction similar?
What is Data Extraction?
Data Extraction is the technique of retrieving and extracting data from various sources for data processing and analyzing purposes. The extracted data may be structured or unstructured data. The extracted data is migrated and stored into a data warehouse from which it is further analyzed and interpreted for business cases.
To make the extraction process more manageable and efficient, Data Engineers make use of Data Extraction tools. When chosen carefully, Data Extraction tools can help companies reap optimal benefits from data. Don’t get confused data extraction tools with data science tools. To get more idea about data extraction, check out our data science online certifications from top universities.
Without further ado, let’s check out some of the most widely used Data Extraction tools!
Top Data Extraction Tools of 2021
1.
is a web-based tool that is used for extracting data from websites. The best part about this tool is that you do not need to write any code for retrieving data – does that by itself. This tool is best suited for equity research, e-commerce and retail, sales and marketing intelligence, and risk management.
The biggest USP of is helping companies achieve success using “smart data” along with data visualization and reporting features. To use this Data Extraction tool, you don’t require any special skills or expertise. It is very user-friendly and hence, accessible to users of all skill levels.
2. OutWit Hub
One of the most extensively used web scraping and Data Extraction tools in the market, OutWit Hub browses the Web and automatically collects and organizes relevant data from online sources. The tool first segregates web pages into separate elements and then navigates them individually to extract the most relevant data from them. It is primarily used for extracting data tables, images, links, email IDs, and much more.
OutWit Hub is a generic tool that packs in a wide range of usage – right from ad hoc data extraction on distinct research topics to performing SEO analysis on websites. It combines a mix of both simple and advanced functions, including web scraping and data structure recognition. OutWit Hub has an extension for both Chrome and Mozilla Firefox.
3. Octoparse
With Octoparse, you can extract data in three simple steps – pointing, clicking, ad extracting – without requiring any code. You just have to enter the website URL you wish to scrape and extract data from, then click on the target data, and finally run the extraction function to retrieve the data! It is that simple.
Octoparse allows you to scrape any website. It uses automatic IP rotation to prevent sites from blocking your IP address. This lets you scrape as many websites as you would like. Besides being extremely user-friendly, Octoparse is laden with many advanced features like a 24/7 cloud platform and scraping scheduler. You can also download the extracted data as CSV, Excel, API files or save them directly to your database.
4. Web Scraper
Just like Octoparse, Web Scraper is another point-and-click Data Extraction tool. As its official website claims, the goal of Web Scraper is “to make web data extraction easy and accessible for everyone. ” Specially designed for the Web, this Data Extraction tool can extract data from any and every website, including those with features like multi-level navigation, JavaScript, or infinite scrolling.
With Web Scraper, you can build site maps from different kinds of selectors which further makes it possible to tailor Data Extraction to disparate site structures. The Cloud Web Scraper service lets you access the extracted data via API or webhooks. Since it has an in-built cloud service, it can scale with your growing business – so you need not worry about outgrowing its services.
Read: Data Engineer Salary in India
5. ParseHub
ParseHub is a popular web scraping and Data Extraction tool that helps you extract relevant data in a few clicks. It can not only scrape complex websites using JavaScript and Ajax, but it can also scrape sites use infinite scrolling or the ones that restrict content with logins.
You simply have to open a website and click the data you want to extract, and that’s it. ParseHub’s ML relationship engine can screen the page/site to understand the hierarchy of elements and hand out the desired data in seconds.
You can download the extracted data in JSON, Excel, or API formats. Also, you can instruct ParseHub to search through forms and maps, open drop downs, login to websites, and handle websites with infinite scroll, tabs, and pop-ups.
6. Mailparser
Mailparser is an advanced email parser that can extract data from emails. Email parsing is different from web scraping in the sense that in email parsing instead of extracting data from HTML websites, the tool pulls data from emails.
MailParser is a powerful and easy-to-use tool that lets you extract data without requiring any elaborate coding. It has an all-round tool – the HTTP Webhook that can perform a wide variety of functions.
To use Mailparser, you need to forward the emails to it, and the tool automatically scrapes the data you want to extract based on the custom extraction rules that you feed in the tool during the set-up process. After the data is retrieved, you can export the scraped data either through file downloads/native integrations or through the generic HTTP Webhooks.
7. DocParser
DocParser is a Data Extraction tool specifically designed to extract data from business documents. This versatile tool makes use of a custom parsing engine that can support numerous and varied use cases. It extracts all the relevant information (data) from business documents and moves it to the desired location.
DocParser completely eliminates the task of manual data entry and streamlines your business with non-disruptive workflow automation. You can use DocParser for processing invoice and accounts payable; converting purchase & sales orders, and HR forms; extract data from standardized contracts and agreements, among other things.
Wrapping Up
These are seven top Data Extraction tools should be on your checklist if you work with Big Data or are aspiring to build a career in this field. The biggest advantage of using Data Extraction tools is that they eliminate the manual factor from the equation, thereby saving both time and money.
If you are curious to learn about data science, check out IIIT-B & upGrad’s Executive PG Programme in Data Science which is created for working professionals and offers 10+ case studies & projects, practical hands-on workshops, mentorship with industry experts, 1-on-1 with industry mentors, 400+ hours of learning and job assistance with top firms.
In how many ways data can be extracted?
Data extraction is the process of gathering data from various sources for analyzing and processing data. This data can be extracted according to the analysis goals and company needs. There are three possible ways to extract data that are as follows. In Update Notification type of extraction, the source system sends a notification whenever a change has been made in a record. Many databases come with similar functionality to support database replication. Incremental Extraction makes the delta changes in the data. The engineer first needs to add complex data extraction logic in the source system before extracting the data. The extraction tools are programmed to detect any changes made, based on the time and date. Some data sources have no mechanism to identify any changes made to the source data. In that case, a full extraction is the only way left to replicate the source.
What are the applications of OutWit Hub?
OutWit Hub is one of the leading data extraction tools and is known for various applications in multiple domains. Some of these applications are as follows – OutWit allows you to extract the latest news from the search engines using its built-in RSS feed extractor. You can use it for SEO purposes as it can monitor the key elements in the websites or even on selected web pages. Deep web searches, social networking monitoring, and e-commerce are some other applications of OutWit Hub.
Are data mining and data extraction similar?
Many people get confused between data mining and data extraction and end up considering them two different terms for the same process. But this is a wrong deduction. Data mining and data extraction are different from each other right from the definition. Data mining is the process where large chunks of data are analyzed to gather some similarities, patterns, or relationships between different data sets that are missed by the traditional analyses techniques. Data extraction on the other hand extracts the data from the online data sources which is stored in the data warehouses for further processing.
PG Diploma in Data Science
A Guide to Evidence Synthesis: 10. Data Extraction
Additional Information
These resources offer additional information and examples of data extraction forms:
Brown, S. A., Upchurch, S. L., & Acton, G. J. (2003). A framework for developing a coding scheme for meta-analysis. Western Journal of Nursing Research, 25(2), 205–222.
Elamin, M. B., Flynn, D. N., Bassler, D., Briel, M., Alonso-Coello, P., Karanicolas, P. J., … Montori, V. M. (2009). Choice of data extraction tools for systematic reviews depends on resources and review complexity. Journal of Clinical Epidemiology, 62(5), 506–510.
Higgins, J. P. T., & Deeks, J. (Eds. ) (2011). Chapter 7: Selecting studies and collecting data. In J. T. Higgins, & S. Green (Eds. ), Cochrane handbook for systematic reviews of interventions Version 5. 1. 0 (updated March 2011). The Cochrane Collaboration. Available from
Research guide from the George Washington University Himmelfarb Health Sciences Library:
Data extraction for ETL simplified | BryteFlow
What is Data Extraction?
Data extraction refers to the method by which organizations get data from databases or SaaS platforms in order to replicate it to a data warehouse or data lake for Reporting, Analytics or Machine Learning purposes. When data is extracted from various sources, it has to be cleaned, merged and transformed into a consumable format and stored in the data repository for querying. This process is the ETL process or Extract Transform Load.
Data Extraction refers to the ‘E’ of the Extract Transform Load process
Data extraction as the name suggests is the first step of the Extract Transform Load sequence. The process of data extraction involves retrieval of data from various data sources. The source of data, which is usually a database, or files, XMLs, JSON, API etc. is crawled through to retrieve relevant information in a specific pattern. Data ETL includes processing which involves adding metadata information and other data integration processes that are all part of the ETL workflow.
The purpose is to prepare and process the data further, migrate the data to a data repository or to further analyse it. In short, to make the most use of the data present. Learn more about BryteFlow for AWS ETL
Why is Data Extraction so important?
In order to achieve big data goals, data extraction becomes the most important step as everything else is going to be derived from the data that is retrieved from the source. Big data is used for everything and anything including decision making, sales trends forecasting, sourcing new customers, customer service enhancement, medical research, optimal cost cutting, Machine Learning, AI and more. If data extraction is not done properly, the data will be flawed. After all, only high quality data leads to high quality insights.
What to keep in mind when preparing for data extraction during data ETL
Impact on the source: Retrieving information from the source may impact the source system/database. The system may slow down and frustrate other users accessing it at the time. This should be thought of when planning for data extraction. The performance of the source system shouldn’t be compromised. You should opt for a data extraction approach that has minimal impact on the source.
Volume: Data extraction involves ingesting large volumes of data which the process should be able to handle efficiently. Analyze the source volume and plan accordingly. Data extraction of large volumes calls for a multi-threaded approach and might also need virtual grouping/partitioning of data into smaller chunks or slices for faster data ingestion.
Secrets of Bulk Loading Data Fast to Cloud Data Warehouses
Data completeness: For continually changing data sources, the extraction approach should cater to capture the changes in data effectively, be it directly from the source or via logs, API, date stamps, triggers etc.
Automated data extraction: let BryteFlow do the heavy hitting
BryteFlow can do all the thinking and planning to get your data extracted smartly for Data Warehouse ETL. Its ticks all the checkboxes above and is very effective in migrating data from any structured/semi-structured sources onto a Cloud DW or Data Lake. Build an S3 Data Lake in Minutes
Types of Data Extraction
Coming back to data extraction, there are two types of data extraction: Logical and Physical extraction.
Logical Extraction
The most commonly used data extraction method is Logical Extraction which is further classified into two categories:
Full Extraction
In this method, data is completely extracted from the source system. The source data will be provided as is and no additional logical information is necessary on the source system. Since it is complete extraction, there is no need to track the source system for changes.
For e. g., exporting a complete table in the form of a flat file.
Incremental Extraction
In incremental extraction, the changes in source data need to be tracked since the last successful extraction. Only these changes in data will be retrieved and loaded. There can be various ways to detect changes in the source system, maybe from the specific column in the source system that has the last changed timestamp. You can also create a change table in the source system, which keeps track of the changes in the source data. It can also be done via logs if the redo logs are available for the rdbms sources. Another method for tracking changes is by implementing triggers in the source database.
Physical Extraction
Physical extraction has two methods: Online and Offline extraction:
Online Extraction
In this process, the extraction process directly connects to the source system and extracts the source data.
Offline Extraction
The data is not extracted directly from the source system but is staged explicitly outside the original source system. You can consider the following common structure in offline extraction:
Flat file: Is in a generic format
Dump file: Database specific file
Remote extraction from database transaction logs
There can be several ways to extract data offline, but the most efficient of them all is to do via remote data extraction from database transaction logs. Database archive logs can be shipped to a remote server where data is extracted. This has zero impact on the source system and is high performing. The extracted data is loaded into a destination that serves as a platform for AI, ML or BI reporting, such as a cloud data warehouse like Amazon Redshift, Azure SQL Data Warehouse or Snowflake. The load process needs to be specific to the destination.
Data extraction with Change Data Capture
Incremental extraction is best done with Change Data Capture or CDC. If you need to extract data regularly from a transactional database that has frequent changes, Change Data Capture is the way to go. With CDC, only the data that has changed since the last data extraction is loaded to the data warehouse not the full refresh which is extremely time-consuming and taxing on resources. Change Data Capture enables access of near real-time data or on-time data warehousing. Change Data Capture is inherently more efficient since a much smaller volume of data needs to be extracted. However mechanisms to identify the recently modified data may be challenging to put in place, that’s where a data extraction tool like BryteFlow can help. It provides automated CDC replication so there is no coding involved and data extraction and replication is super-fast even from traditional legacy databases like SAP and Oracle.
Automated Data Extraction with BryteFlow for Data Warehouse ETL
BryteFlow uses a remote extraction process with Change Data Capture and provides automated data replication with:
Zero impact on source
High performance: multi threaded configurable extraction and loading and provides the highest throughput in the market when compared with competitors
Zero coding: for extraction, merging, masking or type 2 history
Support for terabytes of data ingestion, both initial and incremental
Time series your data
Self-recovery from connection dropouts
Smart catch-up features in case of down-time
CDC with Transaction Log Replication
Automated Data Reconciliation to check for Data Completeness
Simplify data extraction and integration with an automated data extraction tool
BryteFlow integrates data from any API, any flat files and from legacy databases like SAP, Oracle, SQL Server, MySQL and delivers ready-to-use data to S3, Redshift, Snowflake, Azure Synapse and SQL Server at super-fast speeds. It is completely self-service, needs no coding, and low maintenance. It can handle huge petabytes of data easily with smart partitioning and parallel multi-thread loading.
BryteFlow is ideal for Data Warehouse ETL
BryteFlow Ingest uses an easy-to-use point and click interface to set up real-time database replication to your destination with high parallelism for the best performance. BryteFlow is secure. It is a cloud-based solution that specializes in securely extracting, transforming, and loading your data. As a part of the data warehouse ETL process, if you need to mask sensitive information or split columns on the fly, it can be done with simple configuration using BryteFlow. Learn how BryteFlow Data Replication Software works
Want to know more about easy real-time data extraction and replication? Get a free trial of BryteFlow
Frequently Asked Questions about data extraction tools
What are the two types of data extraction?
Coming back to data extraction, there are two types of data extraction: Logical and Physical extraction.
What are the data extraction techniques?
Data can be extracted in three primary ways:Update notification. The easiest way to extract data from a source system is to have that system issue a notification when a record has been changed. … Incremental extraction. … Full extraction. … API-specific challenges.
What is big data extraction tools?
Data extraction tools efficiently and effectively read various systems, such as databases, ERPs, and CRMs, and collect the appropriate data found within each source. Most tools have the ability to gather any data, whether structured, semi-structured, or unstructured.