…
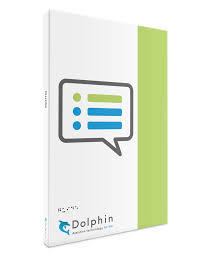
Building A Web Crawler
How to build a web crawler? – Scraping-bot.io
At the era of big data, web scraping is a life saver. To save even more time, you can couple ScrapingBot to a web crawling bot.
What is a web crawler?
A crawler, or spider, is an internet bot indexing and visiting every URLs it encounters. Its goal is to visit a website from end to end, know what is on every webpage and be able to find the location of any information. The most known web crawlers are the search engine ones, the GoogleBot for example. When a website is online, those crawlers will visit it and read its content to display it in the relevant search result pages.
How does a web crawler work?
Starting from the root URL or a set of entries, the crawler will fetch the webpages and find other URLs to visit, called seeds, in this page. All the seeds found on this page will be added on its list of URLs to be visited. This list is called the horizon. The crawler organises the links in two threads: ones to visit, and already visited ones. It will keep visiting the links until the horizon is empty.
Because the list of seeds can be very long, the crawler has to organise those following several criterias, and prioritise which ones to visit first and revisit. To know which pages are more important to crawl, the bot will consider how many links go to this URL, how often it is visited by regular users.
What is the difference between a web scraper and a web crawler?
Crawling, by definition, always implies the web. A crawler’s purpose is to follow links to reach numerous pages and analyze their meta data and content.
Scraping is possible out of the web. For example you can retrieve some information from a database. Scraping is pulling data from the web or a database.
Why do you need a web crawler?
With web scraping, you gain a huge amount of time, by automatically retrieving the information you need instead of looking for it and copying it manually. However, you still need to scrape page after page. Web crawling allows you to collect, organize and visit all the pages present on the root page, with the possibility to exclude some links. The root page can be a search result or category.
For example, you can pick a product category or a search result page from amazon as an entry, and crawl it to scrape all the product details, and limit it to the first 10 pages with the suggested products as well.
How to build a web crawler?
The first thing you need to do is threads:
Visited URLsURLs to be visited (queue)
To avoid crawling the same page over and over, the URL needs to automatically move to the visited URLs thread once you’ve finished crawling it. In each webpage, you will find new URLs. Most of them will be added to the queue, but some of them might not add any value for your purpose. Hence why you also need to set rules for URLs you’re not interested in.
Deduplication is a critical part of web crawling. On some websites, and particularly on e-commerce ones, a single webpage can have multiple URLs. As you want to scrape this page only once, the best way to do so is to look for the canonical tag in the code. All the pages with the same content will have this common canonical URL, and this is the only link you will have to crawl and scrape.
Here’s an example of a canonical tag in HTML:
previousDepth) {
previousDepth =;
(`——- CRAWLING ON DEPTH LEVEL ${previousDepth} ——–`);}
return nextLink;}
function peekInQueue() {
return linksQueue[0];}
//Adds links we’ve visited to the seenList
function addToSeen(linkObj) {
seenLinks[] = linkObj;}
//Returns whether the link has been seen.
function linkInSeenListExists(linkObj) {
return seenLinks[] == null? false: true;}
Is web crawling legal? – Towards Data Science
Photo by Sebastian Pichler on UnsplashWeb crawling, also known as web scraping, data scraping or spider, is a computer program technique used to scrape a huge amount of data from websites where regular-format data can be extracted and processed into easy-to-read structured crawling basically is how the internet functions. For example, SEO needs to create sitemaps and gives their permissions to let Google crawl their sites in order to make higher ranks in the search results. Many consultant companies would hire companies to specialize in web scraping to enrich their database so as to provide professional service to their is really hard to determine the legality of web scraping in the era of the digitized crawling can be used in the malicious purpose for example:Scraping private or classified information. Disregard of the website’s terms and service, scrape without owners’ abusive manner of data requests would lead web server crashes under additionally heavy is important to note that a responsible data service provider would refuse your request if:The data is private which would need a username and passcodesThe TOS (Terms of Service) explicitly prohibits the action of web scrapingThe data is copyrightedViolation of the Computer Fraud and Abuse Act (CFAA). Violation of the Digital Millennium Copyright Act (DMCA)Trespass to “just scraped a website” may cause unexpected consequences if you used it probably heard of the HiQ vs Linkedin case in 2017. HiQ is a data science company that provides scraped data to corporate HR departments. Linkedin then sent desist letter to stop HiQ scraping behavior. HiQ then filed a lawsuit to stop Linkedin from blocking their access. As a result, the court ruled in favor of HiQ. It is because that HiQ scrapes data from the public profiles on Linkedin without logging in. That said, it is perfectly legal to scrape the data which is publicly shared on the ’s take another example to illustrate in what case web scraping can be harmful. The law case eBay v. Bidder’s Edge. If you’re doing web crawling for your own purposes, it is legal as it falls under fair use doctrine. The complications start if you want to use scraped data for others, especially commercial purposes. Quoted from, 100 1058 (N. D. Cal. 2000), was a leading case applying the trespass to chattels doctrine to online activities. In 2000, eBay, an online auction company, successfully used the ‘trespass to chattels’ theory to obtain a preliminary injunction preventing Bidder’s Edge, an auction data aggregation, from using a ‘crawler’ to gather data from eBay’s website. The opinion was a leading case applying ‘trespass to chattels’ to online activities, although its analysis has been criticized in more recent long as you are not crawling at a disruptive rate and the source is public you should be fine. I suggest you check the websites you plan to crawl for any Terms of Service clauses related to scraping their intellectual property. If it says “no scraping or crawling”, you should respect ggestion:Scrape discreetly, check “” before you start scrapingGo conservative. Aggressively asking for data can burden the internet server. An ethical way is to be gentle. No one wants to crash the the data wisely. Don’t duplicate the data. You can generate insight from collected data, and help Your business out to the owner of the website before you start ’t randomly pass scraped data to anyone. If it is valuable data, keep it secure.
How To Crawl A Web Page with Scrapy and Python 3 – DigitalOcean
Introduction
Web scraping, often called web crawling or web spidering, or “programmatically going over a collection of web pages and extracting data, ” is a powerful tool for working with data on the web.
With a web scraper, you can mine data about a set of products, get a large corpus of text or quantitative data to play around with, get data from a site without an official API, or just satisfy your own personal curiosity.
In this tutorial, you’ll learn about the fundamentals of the scraping and spidering process as you explore a playful data set. We’ll use BrickSet, a community-run site that contains information about LEGO sets. By the end of this tutorial, you’ll have a fully functional Python web scraper that walks through a series of pages on Brickset and extracts data about LEGO sets from each page, displaying the data to your screen.
The scraper will be easily expandable so you can tinker around with it and use it as a foundation for your own projects scraping data from the web.
Prerequisites
To complete this tutorial, you’ll need a local development environment for Python 3. You can follow How To Install and Set Up a Local Programming Environment for Python 3 to configure everything you need.
Step 1 — Creating a Basic Scraper
Scraping is a two step process:
You systematically find and download web pages.
You take those web pages and extract information from them.
Both of those steps can be implemented in a number of ways in many languages.
You can build a scraper from scratch using modules or libraries provided by your programming language, but then you have to deal with some potential headaches as your scraper grows more complex. For example, you’ll need to handle concurrency so you can crawl more than one page at a time. You’ll probably want to figure out how to transform your scraped data into different formats like CSV, XML, or JSON. And you’ll sometimes have to deal with sites that require specific settings and access patterns.
You’ll have better luck if you build your scraper on top of an existing library that handles those issues for you. For this tutorial, we’re going to use Python and Scrapy to build our scraper.
Scrapy is one of the most popular and powerful Python scraping libraries; it takes a “batteries included” approach to scraping, meaning that it handles a lot of the common functionality that all scrapers need so developers don’t have to reinvent the wheel each time. It makes scraping a quick and fun process!
Scrapy, like most Python packages, is on PyPI (also known as pip). PyPI, the Python Package Index, is a community-owned repository of all published Python software.
If you have a Python installation like the one outlined in the prerequisite for this tutorial, you already have pip installed on your machine, so you can install Scrapy with the following command:
pip install scrapy
If you run into any issues with the installation, or you want to install Scrapy without using pip, check out the official installation docs.
With Scrapy installed, let’s create a new folder for our project. You can do this in the terminal by running:
mkdir brickset-scraper
Now, navigate into the new directory you just created:
cd brickset-scraper
Then create a new Python file for our scraper called We’ll place all of our code in this file for this tutorial. You can create this file in the terminal with the touch command, like this:
touch
Or you can create the file using your text editor or graphical file manager.
We’ll start by making a very basic scraper that uses Scrapy as its foundation. To do that, we’ll create a Python class that subclasses, a basic spider class provided by Scrapy. This class will have two required attributes:
name — just a name for the spider.
start_urls — a list of URLs that you start to crawl from. We’ll start with one URL.
Open the file in your text editor and add this code to create the basic spider:
import scrapy
class BrickSetSpider():
name = “brickset_spider”
start_urls = [”]
Let’s break this down line by line:
First, we import scrapy so that we can use the classes that the package provides.
Next, we take the Spider class provided by Scrapy and make a subclass out of it called BrickSetSpider. Think of a subclass as a more specialized form of its parent class. The Spider subclass has methods and behaviors that define how to follow URLs and extract data from the pages it finds, but it doesn’t know where to look or what data to look for. By subclassing it, we can give it that information.
Then we give the spider the name brickset_spider.
Finally, we give our scraper a single URL to start from:. If you open that URL in your browser, it will take you to a search results page, showing the first of many pages containing LEGO sets.
Now let’s test out the scraper. You typically run Python files by running a command like python path/to/ However, Scrapy comes with its own command line interface to streamline the process of starting a scraper. Start your scraper with the following command:
scrapy runspider
You’ll see something like this:
Output2016-09-22 23:37:45 [scrapy] INFO: Scrapy 1. 1. 2 started (bot: scrapybot)
2016-09-22 23:37:45 [scrapy] INFO: Overridden settings: {}
2016-09-22 23:37:45 [scrapy] INFO: Enabled extensions:
[‘scrapy. extensions. logstats. LogStats’,
”,
‘reStats’]
2016-09-22 23:37:45 [scrapy] INFO: Enabled downloader middlewares:
[‘tpAuthMiddleware’,…
”]
2016-09-22 23:37:45 [scrapy] INFO: Enabled spider middlewares:
[‘tpErrorMiddleware’,…
2016-09-22 23:37:45 [scrapy] INFO: Enabled item pipelines:
[]
2016-09-22 23:37:45 [scrapy] INFO: Spider opened
2016-09-22 23:37:45 [scrapy] INFO: Crawled 0 pages (at 0 pages/min), scraped 0 items (at 0 items/min)
2016-09-22 23:37:45 [scrapy] DEBUG: Telnet console listening on 127. 0. 1:6023
2016-09-22 23:37:47 [scrapy] DEBUG: Crawled (200)
2016-09-22 23:37:47 [scrapy] INFO: Closing spider (finished)
2016-09-22 23:37:47 [scrapy] INFO: Dumping Scrapy stats:
{‘downloader/request_bytes’: 224,
‘downloader/request_count’: 1,…
‘scheduler/enqueued/memory’: 1,
‘start_time’: time(2016, 9, 23, 6, 37, 45, 995167)}
2016-09-22 23:37:47 [scrapy] INFO: Spider closed (finished)
That’s a lot of output, so let’s break it down.
The scraper initialized and loaded additional components and extensions it needed to handle reading data from URLs.
It used the URL we provided in the start_urls list and grabbed the HTML, just like your web browser would do.
It passed that HTML to the parse method, which doesn’t do anything by default. Since we never wrote our own parse method, the spider just finishes without doing any work.
Now let’s pull some data from the page.
We’ve created a very basic program that pulls down a page, but it doesn’t do any scraping or spidering yet. Let’s give it some data to extract.
If you look at the page we want to scrape, you’ll see it has the following structure:
There’s a header that’s present on every page.
There’s some top-level search data, including the number of matches, what we’re searching for, and the breadcrumbs for the site.
Then there are the sets themselves, displayed in what looks like a table or ordered list. Each set has a similar format.
When writing a scraper, it’s a good idea to look at the source of the HTML file and familiarize yourself with the structure. So here it is, with some things removed for readability:
…
Scraping this page is a two step process:
First, grab each LEGO set by looking for the parts of the page that have the data we want.
Then, for each set, grab the data we want from it by pulling the data out of the HTML tags.
scrapy grabs data based on selectors that you provide. Selectors are patterns we can use to find one or more elements on a page so we can then work with the data within the element. scrapy supports either CSS selectors or XPath selectors.
We’ll use CSS selectors for now since CSS is the easier option and a perfect fit for finding all the sets on the page. If you look at the HTML for the page, you’ll see that each set is specified with the class set. Since we’re looking for a class, we’d use for our CSS selector. All we have to do is pass that selector into the response object, like this:
def parse(self, response):
SET_SELECTOR = ”
for brickset in (SET_SELECTOR):
pass
This code grabs all the sets on the page and loops over them to extract the data. Now let’s extract the data from those sets so we can display it.
Another look at the source of the page we’re parsing tells us that the name of each set is stored within an h1 tag for each set:
Brick Bank
10251-1
The brickset object we’re looping over has its own css method, so we can pass in a selector to locate child elements. Modify your code as follows to locate the name of the set and display it:
NAME_SELECTOR = ‘h1::text’
yield {
‘name’: (NAME_SELECTOR). extract_first(), }
Note: The trailing comma after extract_first() isn’t a typo. We’re going to add more to this section soon, so we’ve left the comma there to make adding to this section easier later.
You’ll notice two things going on in this code:
We append::text to our selector for the name. That’s a CSS pseudo-selector that fetches the text inside of the a tag rather than the tag itself.
We call extract_first() on the object returned by (NAME_SELECTOR) because we just want the first element that matches the selector. This gives us a string, rather than a list of elements.
Save the file and run the scraper again:
This time you’ll see the names of the sets appear in the output:
Output…
[scrapy] DEBUG: Scraped from <200 >
{‘name’: ‘Brick Bank’}
{‘name’: ‘Volkswagen Beetle’}
{‘name’: ‘Big Ben’}
{‘name’: ‘Winter Holiday Train’}…
Let’s keep expanding on this by adding new selectors for images, pieces, and miniature figures, or minifigs that come with a set.
Take another look at the HTML for a specific set:
…
The brickset object we’re looping over has its own css method, so we can pass in a selector to locate child elements. Modify your code as follows to locate the name of the set and display it:
NAME_SELECTOR = ‘h1::text’
yield {
‘name’: (NAME_SELECTOR). extract_first(), }
Note: The trailing comma after extract_first() isn’t a typo. We’re going to add more to this section soon, so we’ve left the comma there to make adding to this section easier later.
You’ll notice two things going on in this code:
We append::text to our selector for the name. That’s a CSS pseudo-selector that fetches the text inside of the a tag rather than the tag itself.
We call extract_first() on the object returned by (NAME_SELECTOR) because we just want the first element that matches the selector. This gives us a string, rather than a list of elements.
Save the file and run the scraper again:
This time you’ll see the names of the sets appear in the output:
Output…
[scrapy] DEBUG: Scraped from <200 >
{‘name’: ‘Brick Bank’}
{‘name’: ‘Volkswagen Beetle’}
{‘name’: ‘Big Ben’}
{‘name’: ‘Winter Holiday Train’}…
Let’s keep expanding on this by adding new selectors for images, pieces, and miniature figures, or minifigs that come with a set.
Take another look at the HTML for a specific set:
10251: Brick Bank
…
We can see a few things by examining this code:
The image for the set is stored in the src attribute of an img tag inside an a tag at the start of the set. We can use another CSS selector to fetch this value just like we did when we grabbed the name of each set.
Getting the number of pieces is a little trickier. There’s a dt tag that contains the text Pieces, and then a dd tag that follows it which contains the actual number of pieces. We’ll use XPath, a query language for traversing XML, to grab this, because it’s too complex to be represented using CSS selectors.
Getting the number of minifigs in a set is similar to getting the number of pieces. There’s a dt tag that contains the text Minifigs, followed by a dd tag right after that with the number.
So, let’s modify the scraper to get this new information:
name = ‘brick_spider’
PIECES_SELECTOR = ‘. //dl[dt/text() = “Pieces”]/dd/a/text()’
MINIFIGS_SELECTOR = ‘. //dl[dt/text() = “Minifigs”]/dd[2]/a/text()’
IMAGE_SELECTOR = ‘img::attr(src)’
‘name’: (NAME_SELECTOR). extract_first(),
‘pieces’: (PIECES_SELECTOR). extract_first(),
‘minifigs’: (MINIFIGS_SELECTOR). extract_first(),
‘image’: (IMAGE_SELECTOR). extract_first(), }
Save your changes and run the scraper again:
Now you’ll see that new data in the program’s output:
Output2016-09-22 23:52:37 [scrapy] DEBUG: Scraped from <200 >
{‘minifigs’: ‘5’, ‘pieces’: ‘2380’, ‘name’: ‘Brick Bank’, ‘image’: ”}
2016-09-22 23:52:37 [scrapy] DEBUG: Scraped from <200 >
{‘minifigs’: None, ‘pieces’: ‘1167’, ‘name’: ‘Volkswagen Beetle’, ‘image’: ”}
{‘minifigs’: None, ‘pieces’: ‘4163’, ‘name’: ‘Big Ben’, ‘image’: ”}
{‘minifigs’: None, ‘pieces’: None, ‘name’: ‘Winter Holiday Train’, ‘image’: ”}
{‘minifigs’: None, ‘pieces’: None, ‘name’: ‘XL Creative Brick Box’, ‘image’: ‘/assets/images/misc/’}
{‘minifigs’: None, ‘pieces’: ‘583’, ‘name’: ‘Creative Building Set’, ‘image’: ”}
Now let’s turn this scraper into a spider that follows links.
Step 3 — Crawling Multiple Pages
We’ve successfully extracted data from that initial page, but we’re not progressing past it to see the rest of the results. The whole point of a spider is to detect and traverse links to other pages and grab data from those pages too.
You’ll notice that the top and bottom of each page has a little right carat (>) that links to the next page of results. Here’s the HTML for that: