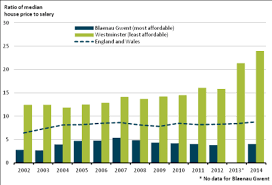
Python Script For Data Scraping
Beautiful Soup: Build a Web Scraper With Python
Watch Now This tutorial has a related video course created by the Real Python team. Watch it together with the written tutorial to deepen your understanding: Web Scraping With Beautiful Soup and Python
The incredible amount of data on the Internet is a rich resource for any field of research or personal interest. To effectively harvest that data, you’ll need to become skilled at web scraping. The Python libraries requests and Beautiful Soup are powerful tools for the job. If you like to learn with hands-on examples and have a basic understanding of Python and HTML, then this tutorial is for you.
In this tutorial, you’ll learn how to:
Inspect the HTML structure of your target site with your browser’s developer tools
Decipher data encoded in URLs
Use requests and Beautiful Soup for scraping and parsing data from the Web
Step through a web scraping pipeline from start to finish
Build a script that fetches job offers from the Web and displays relevant information in your console
Working through this project will give you the knowledge of the process and tools you need to scrape any static website out there on the World Wide Web. You can download the project source code by clicking on the link below:
Let’s get started!
What Is Web Scraping?
Web scraping is the process of gathering information from the Internet. Even copying and pasting the lyrics of your favorite song is a form of web scraping! However, the words “web scraping” usually refer to a process that involves automation. Some websites don’t like it when automatic scrapers gather their data, while others don’t mind.
If you’re scraping a page respectfully for educational purposes, then you’re unlikely to have any problems. Still, it’s a good idea to do some research on your own and make sure that you’re not violating any Terms of Service before you start a large-scale project.
Reasons for Web Scraping
Say you’re a surfer, both online and in real life, and you’re looking for employment. However, you’re not looking for just any job. With a surfer’s mindset, you’re waiting for the perfect opportunity to roll your way!
There’s a job site that offers precisely the kinds of jobs you want. Unfortunately, a new position only pops up once in a blue moon, and the site doesn’t provide an email notification service. You think about checking up on it every day, but that doesn’t sound like the most fun and productive way to spend your time.
Thankfully, the world offers other ways to apply that surfer’s mindset! Instead of looking at the job site every day, you can use Python to help automate your job search’s repetitive parts. Automated web scraping can be a solution to speed up the data collection process. You write your code once, and it will get the information you want many times and from many pages.
In contrast, when you try to get the information you want manually, you might spend a lot of time clicking, scrolling, and searching, especially if you need large amounts of data from websites that are regularly updated with new content. Manual web scraping can take a lot of time and repetition.
There’s so much information on the Web, and new information is constantly added. You’ll probably be interested in at least some of that data, and much of it is just out there for the taking. Whether you’re actually on the job hunt or you want to download all the lyrics of your favorite artist, automated web scraping can help you accomplish your goals.
Challenges of Web Scraping
The Web has grown organically out of many sources. It combines many different technologies, styles, and personalities, and it continues to grow to this day. In other words, the Web is a hot mess! Because of this, you’ll run into some challenges when scraping the Web:
Variety: Every website is different. While you’ll encounter general structures that repeat themselves, each website is unique and will need personal treatment if you want to extract the relevant information.
Durability: Websites constantly change. Say you’ve built a shiny new web scraper that automatically cherry-picks what you want from your resource of interest. The first time you run your script, it works flawlessly. But when you run the same script only a short while later, you run into a discouraging and lengthy stack of tracebacks!
Unstable scripts are a realistic scenario, as many websites are in active development. Once the site’s structure has changed, your scraper might not be able to navigate the sitemap correctly or find the relevant information. The good news is that many changes to websites are small and incremental, so you’ll likely be able to update your scraper with only minimal adjustments.
However, keep in mind that because the Internet is dynamic, the scrapers you’ll build will probably require constant maintenance. You can set up continuous integration to run scraping tests periodically to ensure that your main script doesn’t break without your knowledge.
An Alternative to Web Scraping: APIs
Some website providers offer application programming interfaces (APIs) that allow you to access their data in a predefined manner. With APIs, you can avoid parsing HTML. Instead, you can access the data directly using formats like JSON and XML. HTML is primarily a way to present content to users visually.
When you use an API, the process is generally more stable than gathering the data through web scraping. That’s because developers create APIs to be consumed by programs rather than by human eyes.
The front-end presentation of a site might change often, but such a change in the website’s design doesn’t affect its API structure. The structure of an API is usually more permanent, which means it’s a more reliable source of the site’s data.
However, APIs can change as well. The challenges of both variety and durability apply to APIs just as they do to websites. Additionally, it’s much harder to inspect the structure of an API by yourself if the provided documentation lacks quality.
The approach and tools you need to gather information using APIs are outside the scope of this tutorial. To learn more about it, check out API Integration in Python.
Scrape the Fake Python Job Site
In this tutorial, you’ll build a web scraper that fetches Python software developer job listings from the Fake Python Jobs site. It’s an example site with fake job postings that you can freely scrape to train your skills. Your web scraper will parse the HTML on the site to pick out the relevant information and filter that content for specific words.
You can scrape any site on the Internet that you can look at, but the difficulty of doing so depends on the site. This tutorial offers you an introduction to web scraping to help you understand the overall process. Then, you can apply this same process for every website you’ll want to scrape.
Throughout the tutorial, you’ll also encounter a few exercise blocks. You can click to expand them and challenge yourself by completing the tasks described there.
Step 1: Inspect Your Data Source
Before you write any Python code, you need to get to know the website that you want to scrape. That should be your first step for any web scraping project you want to tackle. You’ll need to understand the site structure to extract the information that’s relevant for you. Start by opening the site you want to scrape with your favorite browser.
Explore the Website
Click through the site and interact with it just like any typical job searcher would. For example, you can scroll through the main page of the website:
You can see many job postings in a card format, and each of them has two buttons. If you click Apply, then you’ll see a new page that contains more detailed descriptions of the selected job. You might also notice that the URL in your browser’s address bar changes when you interact with the website.
Decipher the Information in URLs
A programmer can encode a lot of information in a URL. Your web scraping journey will be much easier if you first become familiar with how URLs work and what they’re made of. For example, you might find yourself on a details page that has the following URL:
You can deconstruct the above URL into two main parts:
The base URL represents the path to the search functionality of the website. In the example above, the base URL is The specific site location that ends with is the path to the job description’s unique resource.
Any job posted on this website will use the same base URL. However, the unique resources’ location will be different depending on what specific job posting you’re viewing.
URLs can hold more information than just the location of a file. Some websites use query parameters to encode values that you submit when performing a search. You can think of them as query strings that you send to the database to retrieve specific records.
You’ll find query parameters at the end of a URL. For example, if you go to Indeed and search for “software developer” in “Australia” through their search bar, you’ll see that the URL changes to include these values as query parameters:
The query parameters in this URL are? q=software+developer&l=Australia. Query parameters consist of three parts:
Start: The beginning of the query parameters is denoted by a question mark (? ).
Information: The pieces of information constituting one query parameter are encoded in key-value pairs, where related keys and values are joined together by an equals sign (key=value).
Separator: Every URL can have multiple query parameters, separated by an ampersand symbol (&).
Equipped with this information, you can pick apart the URL’s query parameters into two key-value pairs:
q=software+developer selects the type of job.
l=Australia selects the location of the job.
Try to change the search parameters and observe how that affects your URL. Go ahead and enter new values in the search bar up top:
Change these values to observe the changes in the URL.
Next, try to change the values directly in your URL. See what happens when you paste the following URL into your browser’s address bar:
If you change and submit the values in the website’s search box, then it’ll be directly reflected in the URL’s query parameters and vice versa. If you change either of them, then you’ll see different results on the website.
As you can see, exploring the URLs of a site can give you insight into how to retrieve data from the website’s server.
Head back to Fake Python Jobs and continue exploring it. This site is a purely static website that doesn’t operate on top of a database, which is why you won’t have to work with query parameters in this scraping tutorial.
Inspect the Site Using Developer Tools
Next, you’ll want to learn more about how the data is structured for display. You’ll need to understand the page structure to pick what you want from the HTML response that you’ll collect in one of the upcoming steps.
Developer tools can help you understand the structure of a website. All modern browsers come with developer tools installed. In this section, you’ll see how to work with the developer tools in Chrome. The process will be very similar to other modern browsers.
In Chrome on macOS, you can open up the developer tools through the menu by selecting View → Developer → Developer Tools. On Windows and Linux, you can access them by clicking the top-right menu button (⋮) and selecting More Tools → Developer Tools. You can also access your developer tools by right-clicking on the page and selecting the Inspect option or using a keyboard shortcut:
Mac: Cmd+Alt+I
Windows/Linux: Ctrl+Shift+I
Developer tools allow you to interactively explore the site’s document object model (DOM) to better understand your source. To dig into your page’s DOM, select the Elements tab in developer tools. You’ll see a structure with clickable HTML elements. You can expand, collapse, and even edit elements right in your browser:
The HTML on the right represents the structure of the page you can see on the left.
You can think of the text displayed in your browser as the HTML structure of that page. If you’re interested, then you can read more about the difference between the DOM and HTML on CSS-TRICKS.
When you right-click elements on the page, you can select Inspect to zoom to their location in the DOM. You can also hover over the HTML text on your right and see the corresponding elements light up on the page.
Click to expand the exercise block for a specific task to practice using your developer tools:
Find a single job posting. What HTML element is it wrapped in, and what other HTML elements does it contain?
Play around and explore! The more you get to know the page you’re working with, the easier it will be to scrape it. However, don’t get too overwhelmed with all that HTML text. You’ll use the power of programming to step through this maze and cherry-pick the information that’s relevant to you.
Step 2: Scrape HTML Content From a Page
Now that you have an idea of what you’re working with, it’s time to start using Python. First, you’ll want to get the site’s HTML code into your Python script so that you can interact with it. For this task, you’ll use Python’s requests library.
Create a virtual environment for your project before you install any external package. Activate your new virtual environment, then type the following command in your terminal to install the external requests library:
$ python -m pip install requests
Then open up a new file in your favorite text editor. All you need to retrieve the HTML are a few lines of code:
import requests
URL = ”
page = (URL)
print()
This code issues an HTTP GET request to the given URL. It retrieves the HTML data that the server sends back and stores that data in a Python object.
If you print the attribute of page, then you’ll notice that it looks just like the HTML that you inspected earlier with your browser’s developer tools. You successfully fetched the static site content from the Internet! You now have access to the site’s HTML from within your Python script.
Static Websites
The website that you’re scraping in this tutorial serves static HTML content. In this scenario, the server that hosts the site sends back HTML documents that already contain all the data that you’ll get to see as a user.
When you inspected the page with developer tools earlier on, you discovered that a job posting consists of the following long and messy-looking HTML:
Senior Python Developer
Payne, Roberts and Davis
Stewartbury, AA